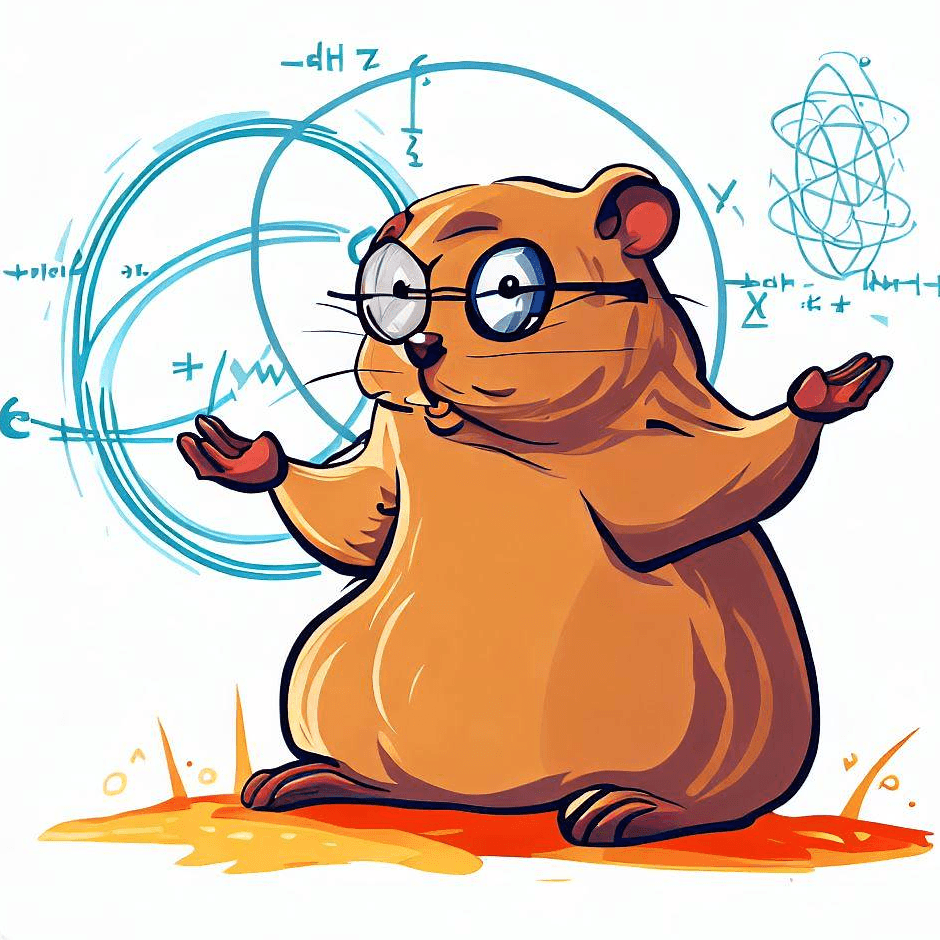
Is the global workspace theory applicable to artificial intelligence and machine learning?
Absolutely! The global workspace theory is like the perfect party dress that fits well on artificial intelligence and machine learning algorithms. π€π It helps them make sense of all the information buzzing around, allowing them to prioritize and focus on important tasks. Just like a superstar DJ at a music festival, AI and machine learning algorithms can dynamically allocate attention to different tasks, based on their relevance and importance. π§πΆπ₯ This way, they can efficiently process and analyze vast amounts of data, making them the life of the data party! πππ₯ So, whether it's recognizing images, translating languages, or playing chess, the global workspace theory provides a framework for AI and machine learning systems to excel! ππ€β¨
- Can you explain further how the global workspace theory helps AI prioritize tasks?
- Can you give examples of how the global workspace theory is applied in machine learning algorithms?
- What are the potential limitations or challenges of applying the global workspace theory to AI systems?
- Could you share some real-world applications where the global workspace theory has been successfully utilized in AI?
- What other theories or frameworks are commonly used in AI and machine learning?
- How does the global workspace theory align with other cognitive theories, such as the attentional bottleneck theory?
- Can you provide more insights on how attention allocation in AI is modeled using the global workspace theory?
- How does the global workspace theory relate to human multitasking abilities?
- Could you elaborate on the concept of the "global workspace" in the context of AI and machine learning?
- Are there any proposed enhancements or modifications to the global workspace theory specific to AI systems?